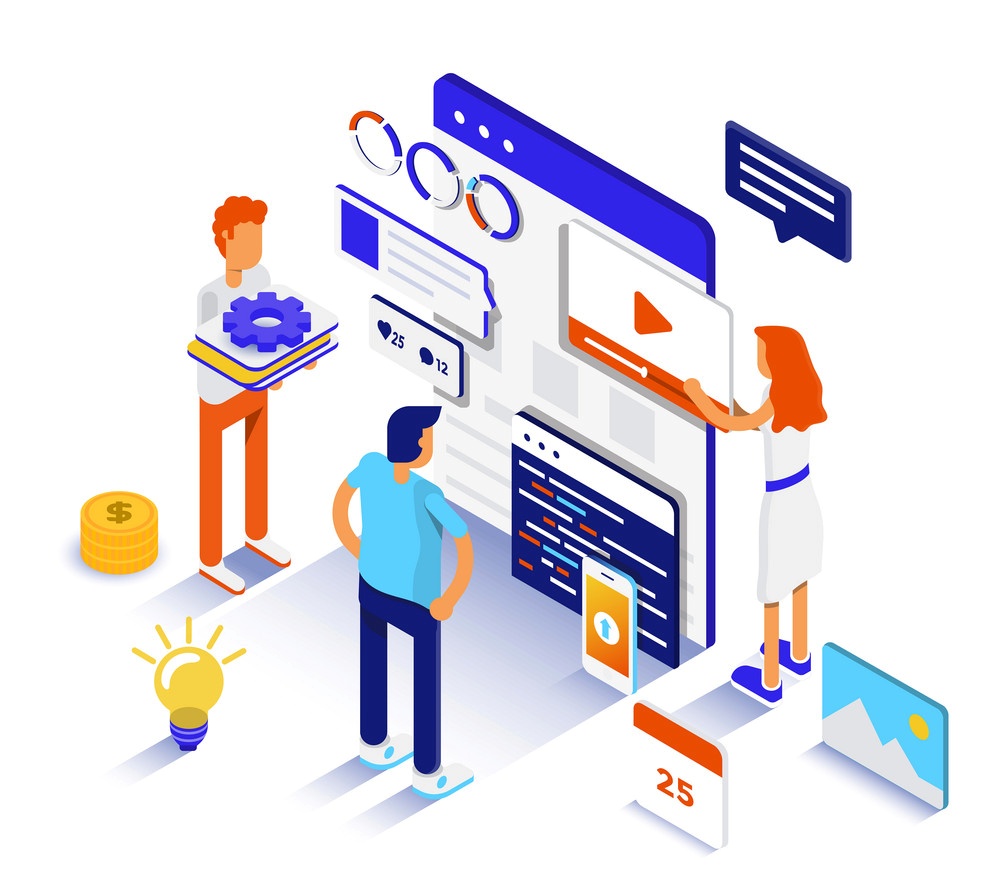
Organizations who want to stride forward quickly in the industry are always keen to make their processes smoother, quicker, and more flexible. In the software industry, software testing remains one critical aspect that companies want to handle as efficiently as possible.
This is where automation testing comes into play. With faster and automated testing procedures, companies can enhance their product development and attain results quicker.
At the same time, it improves testing efficiency because you can detect more problems in a shorter time.
But even when automation testing has so many benefits, it’s still quite challenging for software companies to implement it in their process line. Why? Find out in this post.
It’s Too Costly to Implement Automated Testing
For a start, automated testing is still an expensive option, and you will need substantial capital to implement an automated testing unit. In addition, it makes it harder for software development teams to present the idea to higher management.
Combining that with a long or unpredictable payback time, it becomes virtually impossible to convince the decision-makers.
Return on investment remains a significant obstacle in implementing automation testing, and development teams may be forced to integrate value adders. For instance, you can expect a positive ROI by integrating automation testing with other software products in the system.
Consider end-to-end testing features such as real-time analytics, application loading time, on-page elements, etc. Having real-time access to these parameters can help deliver solutions much faster and more accurately.
Deterministic and Non-Deterministic Testing
Automation testing, when integrated with AI, can produce high-quality results. It’s also called non-deterministic testing because it’s a form of limitless testing. Unlike human-based or deterministic testing, continuous testing involves rigorous models to test applications more comprehensively through AI.
But when businesses only use deterministic testing, they are likely to end in limitless testing cycles and face challenges like exhaustive testing, priority defects, same defects, etc.
Therefore, overcoming challenges with deterministic testing is possible through AI and ML models that can mimic how humans think and act.
Open Source Structure Migration
Open-source solutions are predominantly used in the industry, primarily because they are more affordable and result-oriented. Instead of focusing on more features, open-source solutions ensure that the users get their desired results.
However, open-source solutions don’t get enough budget allocation, so developers don’t get a decent share for their contribution to the project. As a result, it’s not in the interest of companies to invest more in open-source software testing through automation.
In many ways, if an organization must upgrade its open source solutions, it needs to allocate more time and money on the projects while considering cost and benefits.
Moreover, it can also involve licensing issues because open source software doesn’t promote monetization from their new branch of tools. Likewise, security concerns are associated with open-source architectures as bad actors can exploit the data and project contents.
Ecosystem Fragmentation
Right now, the testing landscape is quite fragmented. It’s because the organizations don’t have consistent testing and integration features. For instance, some testing platforms are pretty easy on the development toolchains but lack integration features for test parameters.
Moreover, many testing environments are dedicated to mobile, browser, desktop, or other specific settings. Therefore, testing teams prefer more consistent solutions and apply them throughout the development lifecycle.
Conclusion
Implementing automation testing has been a challenge for organizations, and there is still time before it becomes a common ingredient in the software industry. But given the advancements in AI and ML models, we can expect automation testing to go mainstream in the next couple of years.